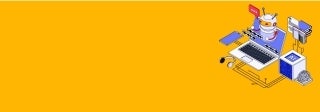
The Impact of AI on (Mis)Information
The Center for Information Networks and Democracy will convene in its 2nd annual workshop a standout lineup of scholars to discuss how AI is transforming the information environment and how we can analyze (and anticipate) the consequences of that transformation.
schedule • abstracts • logistics |
Schedule
Please complete this form if you would like to attend the workshop. Seats are limited and reserved for Penn community members. We will confirm participation via email.
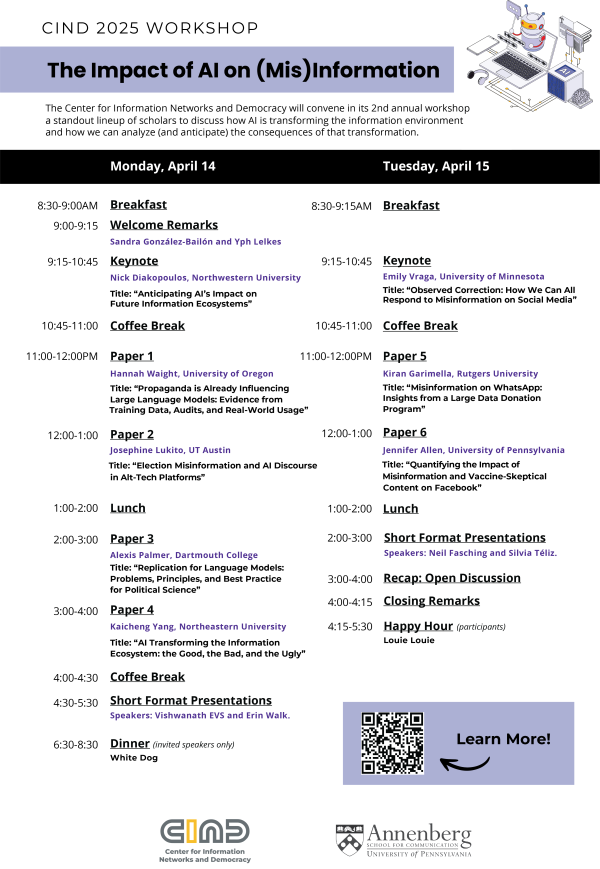
Monday, April 14
- 8:30-9:00am | Breakfast (workshop participants)
- 9:00-9:15 | Welcome remarks
- 9:15-10:45 | Keynote: Nick Diakopoulos, Northwestern University, "Anticipating AI's Impact on Future Information Ecosystems."
- 10:45-11:00 | Coffee break (workshop participants)
- 11:00-12:00pm | Paper 1: Hannah Waight, University of Oregon, "Propaganda is Already Influencing Large Language Models: Evidence from Training Data, Audits, and Real-World Usage."
- 12:00-1:00 | Paper 2: Jo Lukito, The University of Texas at Austin, "Election Misinformation and AI Discourse in Alt-Tech Platforms."
- 1:00-2:00 | Lunch (workshop participants)
- 2:00-3:00 | Paper 3: Alexis Palmer, Dartmouth College, "Replication for Large Language Models: Problems, Principles, and Best Practice for Political Science."
- 3:00-4:00 | Paper 4: Kaicheng Yang, Northeastern University, "AI Transforming the Information Ecosystem: the Good, the Bad, the Ugly."
- 4:00-4:30 | Coffee break (workshop participants)
- 4:30-5:30 | Short Format Presentations, Speakers: Vishwanath EVS, Annenberg School for Communication, University of Pennsylvania, "An LLM-mediated approach to correcting out-group misperceptions and animosity" and Erin Walk, Annenberg School for Communication, University of Pennsylvania, "Attack Rhetoric and Strategy by U.S. Politicians".
- 6:30-8:30 | Dinner at the White Dog (invited speakers only)
Tuesday, April 15
- 8:30-9:15am | Breakfast (workshop participants)
- 9:15-10:45 | Keynote: Emily Vraga, University of Minnesota, "Observed Correction: How We can All Respond to Misinformation on Social Media."
- 10:45-11:00 | Coffee break (workshop participants)
- 11:00-12:00pm | Paper 5: Kiran Garimella, Rutgers University, "Misinformation on WhatsApp: Insights from a Large Data Donation Program."
- 12:00-1:00 | Paper 6: Jennifer Allen, University of Pennsylvania, "Quantifying the Impact of Misinformation and Vaccine-Skeptical Content on Facebook."
- 1:00-2:00 | Lunch (workshop participants)
- 2:00-3:00 | Short Format Presentations, Speakers: Neil Fasching, Annenberg School for Communication, University of Pennsylvania, "Model-Dependent Moderation: Inconsistencies in Hate Speech Detection Across LLM-based Systems" and Silvia Téliz, Annenberg School for Communication, University of Pennsylvania, "A Picture is Worth a Thousand Fictions: Visual Political Communication in the Age of Generative AI".
- 3:00-4:00 | Recap: Open Discussion.
- 4:00-4:15 | Closing remarks
- 4:15-5:30 | Happy Hour at Louie Louie (workshop participants)
Talks
"Replication for Language Models: Problems, Principles, and Best Practice for Political Science", by Alexis Palmer (joint work with Christopher Barrie and Arthur Spirling)
Excitement about Large Language Models (LMs) abounds. These tools require minimal researcher input and yet make it possible to annotate and generate large quantities of data. While LMs are promising, there has been almost no systematic research into the reproducibility of research using them. This is a potential problem for scientific integrity. We give a theoretical framework for replication in the discipline and show that much LM work is wanting. We demonstrate the problem empirically using a rolling iterated replication design in which we compare crowdsourcing and LMs on multiple repeated tasks, over many months. We find that LMs can be (very) accurate, but the observed variance in performance is often unacceptably high. In many cases the LM findings cannot be re-run, let alone replicated. This affects "downstream" results. We conclude with recommendations for best practice, including the use of locally versioned "open source" LMs. [Paper]
"Observed correction: How we can all respond to misinformation on social media", by Emily Vraga
People often criticize social media for facilitating the spread of misinformation. Observed correction, which occurs when direct public corrections of misinformation are witnessed by others, is one important way to combat misinformation because it gives people a more accurate understanding of the topic, especially when they remember the corrections. However, many people—social media users, public health experts, and fact checkers among them—are conflicted or constrained correctors; they think correction is valuable and want to do it well, even as they raise real concerns about the risks and downsides of doing so. Fortunately, simple messages addressing these concerns can make people more willing to respond to misinformation, although addressing other concerns will require changes to the structure of social media and to social norms. Experts, platforms, users, and policymakers all have a role to play to enhance the value of observed correction, which can be an important tool in the fight against misinformation if more people are willing to do it. [Book]
"Attack Rhetoric and Strategy by U.S. Politicians", by Erin Walk
Political elites play a crucial role in shaping public opinion in the U.S., as citizens often rely on elite cues to interpret political events. Affective polarization has been linked to the strategic use of divisive rhetoric by political elites, particularly in electoral contexts. However, existing research tends to emphasize language valence or specific rhetorical forms—such as hate speech and moral appeals—without systematically analyzing the full spectrum of polarizing discourse. A more granular examination of rhetorical strategies is necessary to determine whether particular forms of discourse exert asymmetric effects across ideological and partisan divides, offering a clearer account of the conditions under which elite rhetoric intensifies polarization. Furthermore, the strategic dimensions of elite attacks, including the selection of attack targets, remain understudied. This study addresses these gaps by systematically analyzing personal attacks by legislators. We categorize attack types—such as character, integrity, and patriotism—and assess their impact on public engagement. Building on this foundation, we construct a network of personal attacks to examine patterns in attack strategy and target selection, shedding light on the broader strategic logic.
"Propaganda is Already Influencing Large Language Models: Evidence from Training Data, Audits, and Real-World usage", by Hannah Waight (joint work with Eddie Yang, Yin Yuan, Solomon Messing, Molly Roberts, Brandon Stewart and Joshua Tucker)
We report on a concerning phenomenon in generative AI systems: coordinated propaganda from political institutions influences the output of large language models (LLMs) via the training data for these models. We present a series of five studies that together provide evidence consistent with the argument that LLMs are already being influenced by state propaganda in the context of Chinese state media. First, we demonstrate that material originating from China's Publicity Department appears in large quantities in Chinese language open-source training datasets. Second, we connect this to commercial LLMs by showing not only that they have memorized sequences that are distinctive of propaganda, but propaganda phrases are memorized at much higher rates than those in other documents. Third, we conduct additional training on an LLM with openly available weights to show that training on Chinese state propaganda generates more positive answers to prompts about Chinese political institutions and leaders---evidence that propaganda itself, not mere differences in culture and language, can be a causal factor behind this phenomenon. Fourth, we document an implication in commercial models---that querying in Chinese generates more positive responses about China's institutions and leaders than the same queries in English. Fifth, we show that this language difference holds in prompts related to Chinese politics created by actual Chinese-speaking users of LLMs. Our results suggest the troubling conclusion that going forward there may be strategic incentives for states and other actors to increase the prevalence of propaganda in the future as generative AI becomes more ubiquitous.
"Quantifying the Impact of Misinformation and Vaccine-Skeptical Content on Facebook”, by Jennifer Allen
Low uptake of the COVID-19 vaccine in the US has been widely attributed to social media misinformation. To evaluate this claim, we introduce a framework combining lab experiments (total N = 18,725), crowdsourcing, and machine learning to estimate the causal effect of 13,206 vaccine-related URLs on the vaccination intentions of US Facebook users (N ≈ 233 million). We estimate that the impact of unflagged content that nonetheless encouraged vaccine skepticism was 46-fold greater than that of misinformation flagged by fact-checkers. Although misinformation reduced predicted vaccination intentions significantly more than unflagged vaccine content when viewed, Facebook users’ exposure to flagged content was limited. In contrast, mainstream media stories highlighting rare deaths after vaccination were not flagged by fact-checkers, but were among Facebook’s most-viewed stories. Our work emphasizes the need to scrutinize factually accurate but potentially misleading content in addition to outright falsehoods. Additionally, we show that fact-checking has only limited efficacy in preventing misinformed decision-making and introduce a novel methodology incorporating crowdsourcing and machine learning to better identify misinforming content at scale. [Paper]
"Election Misinformation and AI Discourse in Alt-Tech Platforms: Opportunities and Challenges for Research", by Jo Lukito
False information about elections, including the sharing of incorrect voting details and misinformation about "election fraud" remains a persistent (albeit niche) issue, especially on platforms with weaker moderation policies and strong partisan community beliefs. In these digital spaces, generative AI (genAI) tools may exacerbate information integrity in two ways: (1) using genAI tools to produce misinformation perceived as real and (2) blaming genAI even when the information is true. Using a sample of data from three alt-tech platforms (Telegram, Patriot.Win, and Truth Social) in the months before the 2024 U.S. Presidential election, we explore the extent to which genAI misinformation or incorrect genAI attribution is pervasive in these digital spaces. In conducting this work, we also highlight and consider research-infrastructural limitations for continued research in political misinformation and AI.
"AI Transforming the Information Ecosystem: The Good, the Bad, and the Ugly", by Kaicheng Yang
The rise of generative AI technologies is reshaping the information ecosystem, encompassing production, dissemination, and consumption. Ensuring online platforms remain safe, fair, and trustworthy requires addressing the emerging challenges and opportunities during the transformations. In this talk, I will present my latest research into these dynamics. For production, I focus on malicious AI-powered social bots that generate human-like information and engage with others automatically (Bad), discussing their behaviors and methods for detection. Regarding dissemination, I analyze the capabilities and biases of large language models (LLMs) as information curators in the AI era (Ugly), focusing on their judgments of information source credibility. On the consumption side, I explore how LLMs can be leveraged to detect misleading textual and visual content and provide fact-checking support (Good). Finally, I will reflect on the broader implications of advancing AI models for the reliability and trustworthiness of the information ecosystem and conclude with future directions. [Paper]
"Misinformation on WhatsApp: Insights from a large data donation program", by Kiran Garimella
This research presents the first comprehensive analysis of problematic content circulation on WhatsApp, focusing on private group messages during the national election in India. Through a large-scale data donation program, we obtained a representative sample of users from Uttar Pradesh, India's most populous state with over 200 million inhabitants. This extensive dataset allowed us to examine the prevalence of misinformation, political propaganda, AI-generated content, and hate speech across thousands of users. Our findings reveal a significant presence of political content, with two concerning trends emerging: widespread circulation of previously debunked misinformation and targeted hate speech against Muslim communities. While AI-generated content was minimal, the persistence of debunked misinformation suggests serious limitations in the reach and effectiveness of fact-checking efforts within these private groups. This study makes several key contributions. First, it provides unprecedented quantitative insights into everyday WhatsApp usage patterns and content sharing behaviors. Second, it highlights unique challenges in moderating end-to-end encrypted platforms. Third, it introduces innovative data donation methodologies and tools for collecting representative samples from traditionally inaccessible platforms. The implications of our research extend beyond WhatsApp, offering valuable insights for developing effective content moderation policies across encrypted communication channels. Our data collection approach can be adapted for studying other platforms, particularly crucial in an environment where API access is increasingly restricted.
"Model-Dependent Moderation: Inconsistencies in Hate Speech Detection Across LLM-based Systems", by Neil Fasching
Content moderation systems powered by Large Language Models (LLMs) show significant variability in hate speech detection. Our comparative analysis of seven leading systems—including dedicated Moderation Endpoints, frontier LLMs, and traditional machine learning approaches—reveals that model selection fundamentally determines what content gets classified as hate speech. Using a novel synthetic dataset of 1.3 million sentences, we found that identical content received markedly different classification values across systems, with variations particularly pronounced when analyzing targeted hate speech. Testing across 125 demographic groups demonstrates these divergences reflect systematic differences in how models establish boundaries around harmful content. We apply these results to real-world data (Twitter and podcast transcripts) to determine if these differences produce significant effects in practical applications.
"An LLM-mediated approach to correcting out-group misperceptions and animosity", by Vishwanath EVS
Research shows that perceptions about out-group members are more likely to be false, exacerbating the perceived differences between groups. Prior work that has sought to correct these misperceptions relies on increasing the number of interactions between in and out group members. However, when groups are formed based on salient, identity-based characteristics, simply increasing group interactions may not be enough and may even be counterproductive. Out-group misperceptions can be further exacerbated when they are formed based on a selective view of the shared history between the two groups. In this paper, we propose an approach that relies on Large Language Models (LLMs) as intermediaries in conversations between in and out group members. Recent research demonstrates that LLMs are capable of durably reducing beliefs based on deep but selective knowledge, and that LLM-mediated inter-group communications are better able to facilitate deliberations. In this project, we propose an experiment to extend this line of work to the context of intergroup perceptions of religious minorities in India, where misperceptions are both caused by and a justification for fomenting communal tension.
"Anticipating AI’s Impact on Future Information Ecosystems", by Nick Diakopoulos
The media and information ecosystems that we all inhabit are rapidly evolving in light of new applications of (generative) AI. Drawing on hundreds of written scenarios envisioning the future of media and information around the world in light of these technological changes, in this talk I will first scope out the space of anticipated impact. From highly-personalized content and political manipulation, to efficiencies in content production, new experiences for consumers, and evolving jobs and ethics for professionals, there is a wide array of implications for individuals, organizations, the media system, and for society more broadly. From this overview I will then elaborate a few areas in more depth where we are designing sociotechnical applications to advance benefits, simulating policy interventions to mitigate risks, and empirically studying the evolution and shape of media as it adjusts to generative AI technologies.
"A Picture is Worth a Thousand Fictions: Visual Political Communication in the Age of Generative AI", by Silvia Téliz
Political actors rely heavily on images to communicate with the public. What happens when any picture imaginable is within the reach of their fingers? So far, we have seen Netanyahu sipping a drink by a pool in "Trump Gaza" and Kamala Harris wearing communist garb, among many other bizarre visuals. As the political use of Generative AI becomes increasingly common, concerns about its risks to democracy heighten. This talk explores the most recent findings on the use of Generative AI in visual political communication, highlighting its impact on democratic institutions and the open avenues for Social Science research.
Logistics
When?
The workshop will take place on April 14-15, 2025.
Where?
Room 500 at the Annenberg School for Communication (please, use the Walnut Street entrance to be directed on how to reach the room).
Travel and Accommodation
We have reserved rooms at The Inn at Penn, across the street from Annenberg. If you need assistance with your travel arrangements, please contact Luisa Jacobsen.
schedule • abstracts • logistics |