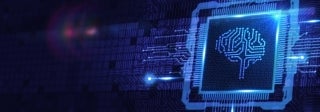
Challenges of AI Implementation: Governance, Ethical Application, Cognition, and Literacy
By Amy Ruckes
One of the most transformative artificial intelligence (AI) technologies, generative AI, emerged in 2022 as the “technology of the year” (Rosenberg, 2022). This emerging technology is a democratizing force because it is applicable to all members of society, including governments, corporations, employees, and individual users. Accordingly, generative AI and generated knowledge production capabilities have fueled demand for “enterprise-wide AI” since it has “grow[n] from a technology employed for particular use cases to one that truly defines the modern enterprise” (MIT, 2023). This heightened demand is especially concerning since AI is “a paradigm-shifting, explosive tool sitting on a powder keg of war and unrest” and is, generally, “operating largely in a regulatory vacuum” (Milton Wolf Seminar, 2024). The panel discussions at the 2024 Milton Wolf Seminar explored the societal implications and uncertainties regarding AI technologies, especially how they will impact the private sector, the public sector, and foreign policy.
It is critical that we address the challenges of safely implementing AI globally since entities are responding to the “hype” of generative AI and rapidly adopting these emerging technologies. Furthermore, the universal applicability of AI means that its implementation impacts businesses, the military, innovation, and education. The competition to hastily embrace new AI technologies might ultimately threaten infrastructure security due to the exposure to increased cyber risks (Humphreys et al., 2024). Cybersecurity threats may develop from human error, data leaks, or malicious activity.
Thus, the initial challenge of AI implementation relates to the exponential growth and adoption of AI technologies, especially the ethical obligations of institutions to safeguard their users, clients, and stakeholders. In March 2024, the European Union passed legislation, the EU Artificial Intelligence Act (AIA), which is one of the most comprehensive attempts to address the societal challenges associated with AI implementation and the “boldest move so far” to regulate AI systems (EU, 2023; Woersdoerfer, 2023). This risk-based regulatory approach requires operators of AI systems to conduct self-assessments. Following this, an appropriate risk category of “unacceptable, high, limited, and minimal“ is assigned to the AI systems and, consequently, regulated according to the risk they pose to society (Novelli et al., 2023).
Although the AIA legislation is specific to Europe, it will establish governance that “applies to both private and public actors inside and outside of the E.U. as long as the AI system impacts E.U. citizens” (Woersdoerfer, 2023, p. 3). Thus, the most outstanding benefit of the AIA is the extra-territorial regulatory effect, referred to as the “Brussels Effect”, which will help establish a global regulatory standard similar to the GDPR. Unfortunately, the risk-based AIA has drawbacks since it gives preference to commercial considerations over ethical concerns, depends on self-assessments, and lacks a legal framework for users/workers to hold providers/employers accountable for human rights abuses (Woersdoerfer, 2023). Interestingly, the AIA is “particularly inadequate for regulating general-purpose AI (GPAI), such as large language models (LLMs) (Novelli et al., 2023, p. 1). Most importantly, the AIA does not regulate AI that is specifically intended for military purposes, national security, or scientific research (ECNL, 2022). Therefore, it is imperative that a more effective and thorough approach to the governance of AI is established globally.
This brings us to the most critical and immediate challenge of AI implementation: the ethical application of AI for military purposes, such as autonomous cyber capabilities, and the need for clearer rules and norms of modern warfare. This was demonstrated most recently during the Israel-Hamas war when the Israel Defense Forces (IDF) used AI-based defense systems known as “Lavender” and “Where’s Daddy?” to automate the command-and-control of identifying and killing Hamas militants in Gaza (Abraham, 2024). Lavender is a targeting system that develops “kill lists” for male Hamas operatives, and Where’s Daddy? is a “home tracking system”. Using both applications together allowed the defense forces to specifically identify and neutralize targets in residential settings during the night “regardless of their rank or military importance”.
The automated targeting system generated a list of 37,000 Palestinians as suspected militants from “visual information, cellular information, social media connections, battlefield information, phone contacts, [and] photos” (Abraham, 2024). Once the targets were identified, the Israeli military personnel were not required to individually check the accuracy of the targeting process, even though there was a known error rate of 10% before the system was fully implemented. In fact, the Israeli military prioritized speed over accuracy, and one commander even viewed the slower processing time from human assessments as a “bottleneck” that limited military operations (Abraham, 2024; Rubenstein, 2023).
Based on mobile phone localization, the residences were marked by the defense system, and the system ordered missiles to attack the suspected Hamas targets, which was often accomplished with unguided missiles several hours after the militant was first tracked to the location. Unguided munitions were often chosen for the attacks and deployed at times when families were asleep at the residences. This increased the number of casualties because these imprecise munitions destroyed entire residential buildings in the attacks. The defense system then estimated the collateral damage casualties based on the assumed number of people still living in the building. The system is “unprecedented” because it permits casualty calculations of “15 or 20 civilians” per “every junior Hamas operative” and “more than 100 civilians” killed during an attack on “a senior Hamas official” (Abraham, 2024). It is important to emphasize that the IDF is achieving military results that are contradictory to the goals of modern military targeting, which aims to maximize accuracy and minimize collateral damage (U.S. Air Force, 2021). This means that the automated weapon systems used by the IDF display all the negative aspects of autonomous weapons, such as not complying with the law of war, not following rules of engagement, and excessive weaponeering failures, including nonoptimal munitions recommendations, high collateral damage considerations, and a lack of commander oversight and accountability.
State actors must be held accountable for the unethical programming of weapon system algorithms that are used against non-state actors, especially when the technology is programmed to target individuals in their homes. Recently, reporting on discussions about bilateral agreements between China and the United States demonstrates that state actors are interested in pledges to ban “AI in autonomous weapons like drones [and] nuclear warhead control” (Deeks, 2023). However, multilateral security agreements are necessary to globally implement ethical and legal restrictions on emerging AI technologies and “war algorithms” to protect vulnerable civilians who might be harmed during both regular and irregular conflicts (Creutz et al., 2024; Schaake, 2024; Vestner & Rossi, 2021). More specifically, a legal review of International Humanitarian Law (IHL) must be conducted for AI-based military applications in the context of the “weapons, means, and methods of warfare according to Article 36 of Additional Protocol I to the 1949 Geneva Conventions” (Vestner & Rossi, 2021, p. 511). Creating stricter laws of war will also impact national rules of engagement (ROE) because ROEs are often “more restrictive than the law of war for a given situation” and “compliance with ROE should guarantee compliance with the law of war” (U.S. Air Force, 2021, p. 71). Unfortunately, while there have been two working groups that discussed cyber security capabilities, they have both failed to tackle the legal risks and ethical threats posed by the implementation of autonomous weapons for offensive and defensive operations (Stroppa, 2023).
Overall, AI-based autonomous cyber capabilities fail because they make decisions based on quantitative data and are unable to process qualitative information. This brings us to the next challenge of AI implementation: the limited ability of cognition and reasoning in generative AI. In an April 2024 interview, Yann LeCun, Meta’s AI Chief, identified four functions related to cognition that differentiate AI intelligence from human intelligence. The four functions that current AI cannot perform are “reasoning, planning, persistent memory, and understanding the physical world” (Macaulay, 2024). Generative AI is currently limited because the systems, especially LLMs, are generally trained on text. The common argument in support of generative AI states that the average human cannot consume the “enormous quantities of data” that generative AI is trained on. However, LeCun points out that “a typical four-year-old has seen 50 times more data than the world’s biggest LLMs” through interactions, visual cues, and auditory information. Thus, “objective-driven AI” needs to be developed and trained on information that mimics human learning to finally help AI achieve abilities similar to human intelligence. This means that the near-future reality might prove to be more mundane than we anticipated because AI cannot replace humans since AI is not yet capable of replicating human cognition. However, AI will cause human behavior to change since emerging AI technologies will convert humans from generators (i.e., producers) of innovation to editors of intelligently designed innovation. As editors, we are required to actively control for errors. This means that our unique human abilities of diverting from pre-determined pathways and identifying, defining, and conceptualizing ethical behavior is an irreplaceable contribution to the safe implementation of AI technologies.
Unfortunately, the discussion on the need for developing clearer rules and norms for autonomous cyber capabilities demonstrates that we are failing to act as editors of AI systems (Schaake, 2024). Possibly, the cumulation of our fears of an uncertain AI-dominated future, an unpredictable labor market, and the delusion of an AI-ruled dystopia have paralyzed us from producing governance that develops a more human-centric AI. These fears seem to be compounded by the anticipation that no effective regulations can properly tackle these societal challenges due to the lengthy, deliberative legislative process and the simultaneous exponential growth of AI technologies. As editors, we must develop ethical guidelines that place humans, rather than technology generators, at the center of AI design and regulation. In fact, “if the broader political and socio-technical impacts on different groups of people are not taken into consideration and operationalized in human-centric technology design and governance, there is a danger that AI services will primarily be built and used to prioritize the needs and interests of technology owners and designers” (Sigfrids et al., 2023, pp. 3-4).
The final challenge of AI implementation is also the best way to address societal fears and anticipation regarding AI as a paradigm-shifting tool: greater AI literacy in schools. Educational agendas must incorporate AI literacy alongside digital literacy because “in addition to knowing and using AI ethically, AI literacy serves as a set of competencies that enables individuals to critically evaluate AI technologies, communicate and collaborate effectively with AI” (Ng et al., 2021, p. 4; Long & Magerko, 2020). Regrettably, the emergence of AI literacy within educational agendas as knowledge-based skills is only a recent phenomenon and still “under-explored” (Long & Magerko, 2020; Ng et al., 2021; Yi, 2021). This means that a lack of AI literacy in educational settings prevents students from being prepared for AI technologies in work environments. Additionally, citizens who lack AI literacy will not be prepared as constituents to advocate for essential AI legislation from their elected representatives. Overall, learning AI skills in educational environments democratizes access to AI, reinforces the benefits of AI-based emerging tools, and provides valuable experiences on the technological affordances of generative AI, such as LLMs (Ng et al., 2021).
We cannot stop AI technologies from heavily impacting employment and jobs due to automation (Gmyrek et al., 2023). Yet, we can begin by requiring ethical considerations to safeguard users, clients, and corporate stakeholders; implementing ethical and legal restrictions for militaries to prevent civilian casualties during violent geopolitical conflicts; and developing human-centric AI technologies that benefit individuals, workers, and society. To ensure that current and future generations are prepared for the challenges of AI, we must also prioritize teaching AI skills in educational institutions.
References
Abraham, Y. (2024, April 3). ‘Lavender’: The AI machine directing Israel’s bombing spree in Gaza. +972 Magazine. https://www.972mag.com/lavender-ai-israeli-army-gaza/.
Cruetz, K., Sinkkonen, V., Javadi, M., Onderco, M. (2024, February 13). The EU and military AI governance: Forging value-based coalitions in an age of strategic competition. Finnish Institute of International Affairs (FIIA). https://www.fiia.fi/en/publication/the-eu-and-military-ai-governance.
Deeks, A. (2023, December 4). Too Much Too Soon: China, the U.S., and Autonomy in Nuclear Command and Control. Lawfare. https://www.lawfaremedia.org/article/too-much-too-soon-china-the-u.s.-and-autonomy-in-nuclear-command-and-control.
ECNL. (2022 March). Scope of the EU Artificial Intelligence Act (AIA): Military Purposes and National Security. European Center for Not-for-Profit Law. https://ecnl.org/sites/default/files/2022-03/ECNL%20Pagers%20on%20scope%20of%20AIA%20ECNL_FINAL.pdf.
EU. (2023, August 6). EU AI Act: first regulation on artificial intelligence. European Parliament. https://www.europarl.europa.eu/topics/en/article/20230601STO93804/eu-ai-act-first-regulation-on-artificial-intelligence.
Gmyrek, P., Berg, J., & Bescond, D. (2023). Generative AI and jobs: A global analysis of potential effects on job quantity and quality. ILO Working Paper, 96.
Humphreys, D., Koay, A., Desmond, D., & Mealy, E. (2024). AI hype as a cyber security risk: the moral responsibility of implementing generative AI in business. AI and Ethics, 1-14. https://link.springer.com/article/10.1007/s43681-024-00443-4.
Long, D., & Magerko, B. (2020, April). What is AI literacy? Competencies and design considerations. In Proceedings of the 2020 CHI conference on human factors in computing systems (pp. 1-16). https://dl.acm.org/doi/pdf/10.1145/3313831.3376727?casa_token=UNfYrCQWhU8AAAAA:c-eO_OqHJFcVUAMlGlbhRWYEIVRtwQoPWBsbG8luAgEgryjf1Vp_524FxJft18LxbjQaMpv8_WnP.
Macaulay, T. (2024, April 10). Meta’s AI chief: LLMs will never reach human-level intelligence. The Next Web. https://thenextweb.com/news/meta-yann-lecun-ai-behind-human-intelligence.
Milton Wolf Seminar. (2024). Bots, Bombs, and Bilateralism: Evolutions in Media and Diplomacy. University of Pennsylvania, Annenberg School for Communication, Milton Wolf Seminar on Media & Diplomacy. https://www.asc.upenn.edu/research/centers/milton-wolf-seminar-media-and-diplomacy/2024-seminar.
MIT. (2023, July 18). The great acceleration: CIO perspectives on generative AI. MIT Technology Review Insights and Databricks. https://www.technologyreview.com/2023/07/18/1076423/the-great-acceleration-cio-perspectives-on-generative-ai/.
Ng, D.T.K., Leung, J.K.L., Chu, S.K.W., & Qiao, M.S. (2021). Conceptualizing AI literacy: An exploratory review. Computers and Education: Artificial Intelligence, 2, 100041. https://www.sciencedirect.com/science/article/pii/S2666920X21000357.
Novelli, C., Casolari, F., Rotolo, A., Taddeo, M., & Floridi, L. (2023). Taking AI risks seriously: a new assessment model for the AI Act. AI & SOCIETY, 1-5. https://link.springer.com/article/10.1007/s00146-023-01723-z.
Rosenberg, L. (2022, December 20). Generative AI: The technology of the year for 2022. BigThink. https://bigthink.com/the-present/generative-ai-technology-of-year-2022/#:~:text=So%2C%20what%20is%20Generative%20AI,dialog%2C%20and%20even%20computer%20code.
Rubenstein, L. (2023, December 21). Israel’s Rewriting of the Law of War. Just Security. https://www.justsecurity.org/90789/israels-rewriting-of-the-law-of-war/.
Schaake, M. (2024, April 30). Military is the missing word in AI safety discussions. Financial Times. https://www.ft.com/content/da03f8e1-0ae4-452d-acd1-ec284b6acd78.
Sigfrids, A., Leikas, J., Salo-Pöntinen, H., & Koskimies, E. (2023). Human-centricity in AI governance: A systemic approach. Frontiers in Artificial Intelligence, 6, 976887. https://www.frontiersin.org/articles/10.3389/frai.2023.976887/full.
Stroppa, M. (2023). Legal and ethical implications of autonomous cyber capabilities: a call for retaining human control in cyberspace. Ethics and Information Technology, 25(1), 7. https://link.springer.com/article/10.1007/s10676-023-09679-w.
U.S. Air Force. (2021, November 21). Air Force Doctrine Publication 3-60, Targeting. United States, Department of Defense, Air Force. https://www.doctrine.af.mil/Portals/61/documents/AFDP_3-60/3-60-AFDP-TARGETING.pdf.
Vestner, T., & Rossi, A. (2021). Legal Reviews of War Algorithms. International Law Studies, 97(1), 26. https://digital-commons.usnwc.edu/cgi/viewcontent.cgi?article=2963&context=ils.
Wörsdörfer, M. (2023). The EU’s artificial intelligence act: an ordoliberal assessment. AI and Ethics, 1-16. https://link.springer.com/article/10.1007/s43681-023-00337-x.
Yi, Y. (2021). Establishing the concept of AI literacy. Jahr–European Journal of Bioethics, 12(2), 353-368. https://hrcak.srce.hr/ojs/index.php/jahr/article/download/20552/11227.