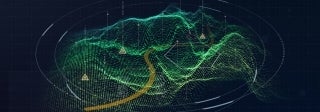
LLMs, Autonomous Weapons, and Human Rights
By Yvonne M. Eadon, Ph.D., MLIS
Topics discussed at the 2024 Milton Wolf Seminar in Vienna, Austria were diverse and operated at a variety of levels—from the speculative and out-of-the-box to the deeply material, historical, and human. On the heels of a grueling exposé of Israel’s use of the “Lavender” system—an AI-powered database—to identify 37,000 Palestinian civilians with purported ties to Hamas , the Seminar saw repeated discussions and provocations about how AI was, is, and will affect the broader landscape of warfare, international relations, and human rights.
The revolution in generative AI in late 2022 with OpenAI’s public launch of ChatGPT seemed to herald a new era. In the ensuing years, there has been a cacophony of utopian and dystopian discourses and predictions about the technology and its applications. On one end, some hail its potential to curb global poverty and provide educational support to students with learning disabilities. On the other, some purport that generative AI is an existential threat to humanity. The promises and pitfalls of generative AI are further complicated by the fact that the models on which generative AI tools are based, Large Language Models (LLMs), require little to no human supervision. Though the details of their capabilities are state secrets, drones used in the Russia-Ukraine conflict appear to be AI weapons that do not require a human to make final decisions about where and when bombs will be dropped.
Researchers have highlighted the ethical and social concerns arising from the broad substitution of human decision-making with AI tools. LLMs are often trained on biased datasets, leading to discriminatory outcomes in various domains such as health, education, criminal justice, and warfare. Research following the 2016 U.S. Presidential Election and BREXIT also marked an important turn in documenting how generative AI can influence voting decisions and impact democratic outcomes.
Most of the research in existence at the moment focuses on text-based applications of LLMs, like generative AI chatbots. LLMs operate based on statistical predictions from user input rather than natural language understanding, making them especially susceptible to bias and manipulation. Malicious users can exploit generative AI tools to alter existing content or produce false information. Models are also susceptible to intentional dataset manipulation through “poisoning attacks.” Model collapse is also becoming an imminent threat as human-generated text is less and less common on the Internet. Some projections suggest that by 2030, 90% of internet content will be computer-generated, potentially leading to generative models spitting out incomprehensible text and ultimately “mis-perceiving reality.” Even today, generative AI chatbots can suffer from hallucinations, generating text divorced from context that results in false answers to queries. This is especially concerning because people consuming AI-generated content often cannot distinguish between the fabricated and the real. This has significant implications for the continued impact of disinformation on our democratic systems—indeed, one panelist at the Seminar suggested that Russia’s weaponization of disinformation as soft power has ushered in a “new Cold War.”
The kind of deep learning that supports generative AI systems has the potential to go in a variety of different directions and be applied to warfare and genocide. Retired IDF general Eyal Zamir told Reuters that AI could facilitate “the ability of platforms to strike in swarms, or of combat systems to operate independently, of data fusion and of assistance in fast decision-making, on a scale greater than we have ever seen.” At the Milton Wolf Seminar, one of the panelists described a similar speculative application of swarm intelligence, in which an automated butterfly could fly in a target’s window and assassinate them, leaving no trace whatsoever. These ideas and speculations are important to think through, as we consider the applications and directions that this technology could take.
The fact that LLMs need no human supervision for generative AI tools to work bodes well for the promise of Autonomous weapons systems (AWS) to accurately identify and destroy military targets without human supervision. These technologies have the potential to completely transform combat and have been heralded by journalist Kai-Fu Lee as “the third revolution in warfare.” United States military has repeatedly claimed that AWS use has “humanitarian benefits” because AWS supposedly allows for more accurate identification and targeting of military objectives. However, the United States, Israel, and other world powers frequently strategically bomb civilians and civilian infrastructure —as we can see unfolding in Israel’s burgeoning offensive on Palestinians it displaced to Rafah.
Underscoring the parallels between nuclear weapons and AI—similarities that were dissected repeatedly by panelists at the Milton Wolf Seminar—Israel has made statements about being an “AI superpower.” The “Lavender” system is just one of many cutting-edge tools that Israel has deployed in its continued assault on Palestinian civilians. “Lavender” is trained on data about confirmed operatives, and then operationalized by analyzing huge corpuses of surveillance data on nearly every single Gazan Palestinian. Its goal in doing so is to rate each individual’s chances of being a Hamas operative. In combination with “Lavender,” Israel is deploying other AI systems. These include “Gospel,” which claims to identify buildings being used by Hamas and puts those higher on a list of places to bomb, and the brutal “Where’s Daddy?”—a system that tracks when suspected Hamas operatives are in their family residences. It is easier for Israel to bomb these family homes than protected buildings, and they frequently do so late at night, when spouses and children are known to be present. These systems are used with scant human oversight, despite their making errors in approximately ten percent of cases—sometimes marking civilians with no connection to Hamas at all.
As AI technology continues to advance at a seemingly exponential rate, International and regional regulatory bodies alike must act quickly and decisively to demand transparency and accountability when it comes to application of the technology. AWS are one more American-made weapon being deployed by Israel in its continued genocide of Palestinians. There are many other applications of AI exhibiting “softer” power that may go unnoticed and unregulated. Regulation of AI is not a novel concept or new demand, the question is, how do we implement it quickly and effectively enough?
References
Bethan McKernan and Harry Davies, “‘The Machine Did It Coldly’: Israel Used AI to Identify 37,000 Hamas Targets,” The Guardian, April 3, 2024, sec. World news, https://www.theguardian.com/world/2024/apr/03/israel-gaza-ai-database-hamas-airstrikes.
Ilia Shumailov et al., “The Curse of Recursion: Training on Generated Data Makes Models Forget” (arXiv, April 14, 2024), http://arxiv.org/abs/2305.17493.
David Adam, “Lethal AI Weapons Are Here: How Can We Control Them?,” Nature 629, no. 8012 (April 23, 2024): 521–23, https://doi.org/10.1038/d41586-024-01029-0.
Buolamwini, Joy, and Timnit Gebru. “Gender Shades: Intersectional Accuracy Disparities in Commercial Gender Classification.” In Proceedings of Machine Learning Research, 81:1–15, 2018; Noble, Safiya Umoja. Algorithms of Oppression: How Search Engines Reinforce Racism. New York, NY: New York University Press, 2018.
Brayne, Sarah. Predict and Surveil: Data, Discretion, and the Future of Policing. Oxford, New York: Oxford University Press, 2020; Eubanks, Virginia. Automating Inequality: How High-Tech Tools Profile, Police, and Punish the Poor. St. Martin’s Publishing Group, 2018.
Kreps, Sarah, and Doug Kriner. “How AI Threatens Democracy.” Journal of Democracy 34, no. 4 (2023): 122–31.
Bender, Emily M., Timnit Gebru, Angelina McMillan-Major, and Shmargaret Shmitchell. “On the Dangers of Stochastic Parrots: Can Language Models Be Too Big? 🦜.” In Proceedings of the 2021 ACM Conference on Fairness, Accountability, and Transparency, 610–23. Virtual Event Canada: ACM, 2021. https://doi.org/10.1145/3442188.3445922.
Zhou, Jiawei, Yixuan Zhang, Qianni Luo, Andrea G Parker, and Munmun De Choudhury. “Synthetic Lies: Understanding AI-Generated Misinformation and Evaluating Algorithmic and Human Solutions.” In Proceedings of the 2023 CHI Conference on Human Factors in Computing Systems, 1–20. CHI ’23. New York, NY, USA: Association for Computing Machinery, 2023. https://doi.org/10.1145/3544548.3581318.
Schuster, Roei, Tal Schuster, Yoav Meri, and Vitaly Shmatikov. “Humpty Dumpty: Controlling Word Meanings via Corpus Poisoning.” In 2020 IEEE Symposium on Security and Privacy (SP), 1295–1313, 2020. https://doi.org/10.1109/SP40000.2020.00115.
Dodge, Jesse, Maarten Sap, Ana Marasović, William Agnew, Gabriel Ilharco, Dirk Groeneveld, Margaret Mitchell, and Matt Gardner. “Documenting Large Webtext Corpora: A Case Study on the Colossal Clean Crawled Corpus.” arXiv, September 30, 2021. https://doi.org/10.48550/arXiv.2104.08758.
Shumailov, Ilia, Zakhar Shumaylov, Yiren Zhao, Yarin Gal, Nicolas Papernot, and Ross Anderson. “The Curse of Recursion: Training on Generated Data Makes Models Forget.” arXiv, May 31, 2023. https://doi.org/10.48550/arXiv.2305.17493; Europol. “Facing Reality?: Law Enforcement and the Challenge of Deepfakes : An Observatory Report from the Europol Innovation Lab.” LU: Europol Innovation Lab, 2022. https://data.europa.eu/doi/10.2813/08370.
Li, Zihao. “The Dark Side of ChatGPT: Legal and Ethical Challenges from Stochastic Parrots and Hallucination.” arXiv, April 21, 2023. https://doi.org/10.48550/arXiv.2304.14347.
Gao, Catherine A., Frederick M. Howard, Nikolay S. Markov, Emma C. Dyer, Siddhi Ramesh, Yuan Luo, and Alexander T. Pearson. “Comparing Scientific Abstracts Generated by ChatGPT to Original Abstracts Using an Artificial Intelligence Output Detector, Plagiarism Detector, and Blinded Human Reviewers.” bioRxiv, December 27, 2022. https://doi.org/10.1101/2022.12.23.521610.
Reuters. “Israel Aims to Be ‘AI Superpower’, Advance Autonomous Warfare.” Reuters, May 22, 2023, sec. Middle East. https://www.reuters.com/world/middle-east/israel-aims-be-ai-superpower-advance-autonomous-warfare-2023-05-22/.
Kai-Fu Lee, “The Third Revolution in Warfare,” The Atlantic, September 11, 2021, https://www.theatlantic.com/technology/archive/2021/09/i-weapons-are-third-revolution-warfare/620013/.
“Defense Primer: U.S. Policy on Lethal Autonomous Weapon Systems” (Washington, D.C: Congressional Research Service, February 1, 2024), https://crsreports.congress.gov.
Branka Marijan, “Autonomous Weapons: The False Promise of Civilian Protection,” Centre for International Governance Innovation, November 28, 2022, https://www.cigionline.org/articles/autonomous-weapons-the-false-promise-of-civilian-protection/.
AlJazeera. “Death Toll in Israeli Attack on Displaced Palestinians in Rafah Rises to 45.” May 27, 2024. https://www.aljazeera.com/news/2024/5/27/dozens-killed-in-israeli-strike-on-rafah-following-hamas-rocket-attacks.
Sigal Samuel, “Some Say AI Will Make War More Humane. Israel’s War in Gaza Shows the Opposite.,” Vox, May 8, 2024, https://www.vox.com/future-perfect/24151437/ai-israel-gaza-war-hamas-artificial-intelligence.
Yuval Abraham, “‘Lavender’: The AI Machine Directing Israel’s Bombing Spree in Gaza,” +972 Magazine, April 3, 2024, https://www.972mag.com/lavender-ai-israeli-army-gaza/.
Reuters. “Gaza Death Toll: How Many Palestinians Has Israel’s Campaign Killed.” May 14, 2024, sec. Middle East. https://www.reuters.com/world/middle-east/gaza-death-toll-how-many-palestinians-has-israels-campaign-killed-2024-05-14/.