Putting the Spotlight on the Gender Gaps in Digital Spaces
Prof. Sandra González-Bailón co-edited a new journal issue quantifying the extent to which gender bias occurs and is perpetuated online and in digital media.
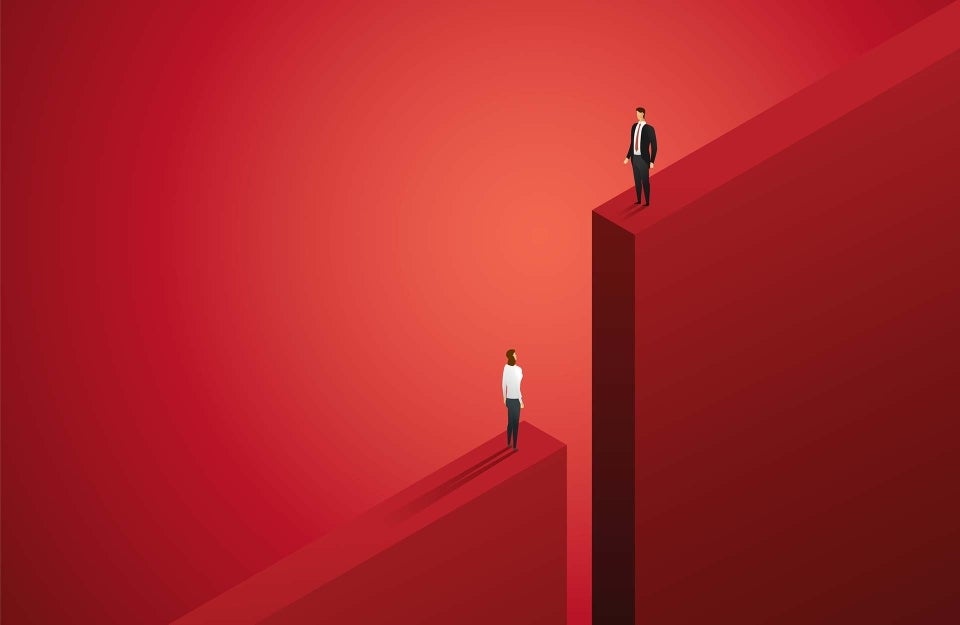
It’s simple to look at a roster of employees at any company and determine if there’s a gender gap. But so many of the systems on which we run our lives — social media platforms, online spaces, recommendation engines built into our media —- are so large and opaque that the task of spotting gender gaps is nearly impossible for the average person.
Enter the computational social scientists.
A new special issue of the Journal of Computer-Mediated Communication, co-edited by Sandra González-Bailón, the Carolyn Marvin Professor of Communication at the Annenberg School for Communication, uncovers how gender gaps exist and perpetuate in online environments. Entitled “Gender Gaps in Digital Spaces,” it includes studies on topics such as gender gaps in political expression, the reality of gender differences in style and quality among programmers, and biases in AI-generated images.
We spoke to González-Bailón about the issue:
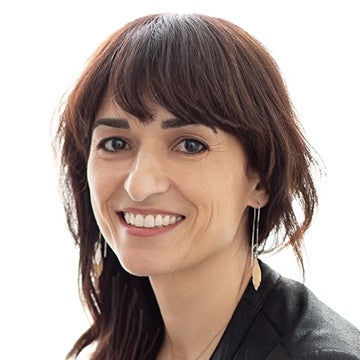
What made you interested in exploring gender disparities online, particularly in a quantitative way?
The idea for this special issue originated during an ICA conference (Paris, 2022). My colleague Brooke Foucault-Welles and I co-organized a panel on how to use computational research to identify gender gaps in digital spaces. The idea was to discuss how we could use empirical evidence to inform interventions that could lessen inequities. In order to make impactful, large-scale changes, you need to begin by measuring where we are now and the trends in these biases. The special issue is a continuation of the discussions we had in that panel.
My co-editor, Ágnes Horvát, and I wanted to energize this area of research, which is particularly crucial as digital technologies evolve rapidly and in unprecedented directions.
What are some examples of the gender divide online?
The digital world is multimodal: you have text, images, videos; you have online games and virtual realities; you have peer-to-peer platforms that facilitate collaboration in the production of outputs (like Wikipedia or GitHub). In all these spaces, there are gendered dynamics and representations that perpetuate stereotypes and bias, and that often create disadvantages for women.
The effect of these biases go beyond the platforms in which they arise; they also have spill-over effects, especially now that Large Language Models and other AI technologies are being trained on untold amounts of existing content, often masking, perpetuating, and even amplifying the biases hidden in those repositories of content.
For example, one of the articles in the special issue explores how AI-generated images for various occupations show more pronounced gender bias than expected, given existing patterns of occupational segregation. It is important that we pay attention to these spillover effects because they often become the unintended consequences that resist correction.
What are some findings that come out of the studies in this issue?
They identify gender differences in three empirical domains: communication dynamics (the way in which mediated interactions contain bias), algorithmic representations (how algorithms perpetuate prejudices), and organizational patterns (how technologies facilitate or hamper collaboration).
They also share a common thread in addressing measurement challenges, in particular, the distorting effects that often result from using binary classifications of gender. One of the articles offers a great assessment of the error that seeps into the research when measurement is not carefully designed. If we want to identify inequities so that we can address them effectively, we first need to get the measurement right.
What are some potential solutions to improve the situation?
In order to create data-driven solutions to gender gaps, we need cumulative, long-term research. The seven articles that form this special issue are a great entry point to the contributions this type of work can make – but we need continuing work to identify the blind spots we may still have when it comes to the creation or perpetuation of gender divides.
For example, in research led by Isabelle Langrock (Ph.D. ‘23), we analyzed efforts to bridge the gender gap on Wikipedia, where only about 20% of the 1.5 million biographical articles are about women. We found that events like “edit-a-thons” to add more articles about women (like the one that Isabelle organized at Annenberg) are effective in narrowing the gap, but that the newly-added articles are also harder to find on the site. Concrete steps, like adding infoboxes, are also necessary to make these new articles more findable.
We need empirical evidence about where the gender gaps exist, in order to pinpoint effective solutions, and the more researchers focus on this, the more solid the evidence becomes. Our hope is that this special issue stimulates more work in this direction.